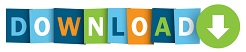
Quantitatively, we apply linear probes to assess whether the network is representing concepts familiar to chess players. We take a quantitative and qualitative approach to interpreting AlphaZero. Our results suggest that it may be possible to understand a neural network using human-defined chess concepts, even when it learns entirely on its own. Furthermore, we observe interesting variance between concepts in terms of when they are learned during training, as well as where in the network’s reasoning chain they are learned. We pair these quantitative results with qualitative analysis from a human world chess champion and compare AlphaZero’s choice of opening over its training to human progress in opening analysis. Although the results are far from a complete understanding of the AlphaZero system, they show evidence for the existence of a broad set of human-understandable concepts within AlphaZero. In this paper, we investigate how AlphaZero represents chess positions and the relation of those representations to human concepts in chess. Although these examples are compelling, each of these networks was exposed to human-generated data and (at least in the case of classifiers) human concepts via the choice of classification categories. Networks may even learn to connect visual and textual versions of the same concept ( 8). Certain language models reproduce sentence parse trees ( 7). Neurons in an image classifier may signal the presence of human concepts in an image ( 4 – 6).

Can we find qualitative or quantitative evidence of such concepts in AlphaZero’s neural network?Įvidence from other domains suggests that deep learning often does produce correlates of human concepts. What exactly has the system learned? Having developed without human input, will it be inevitably opaque? Can its training history shed light on human progress in chess? A human player naturally picks up basic concepts while playing: that a queen is worth more than a pawn or that a check to the king is important. The success of an entirely self-taught system raises intriguing questions. AlphaZero reliably won games not just against top human players but also, against the previous generation of chess engines. The first such engine, the AlphaZero ( 3) system, has at its core an artificial neural network that is trained entirely through self-play.
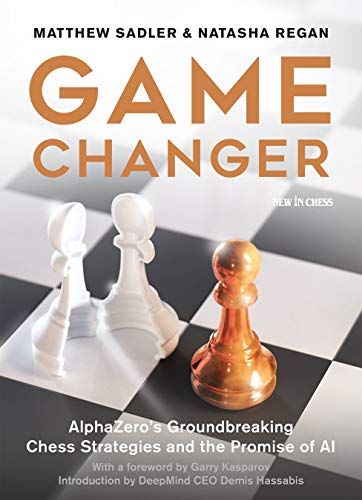
By contrast, a new generation has appeared of highly successful chess engines that learn to play chess without using any human-crafted heuristics or even seeing a human game.
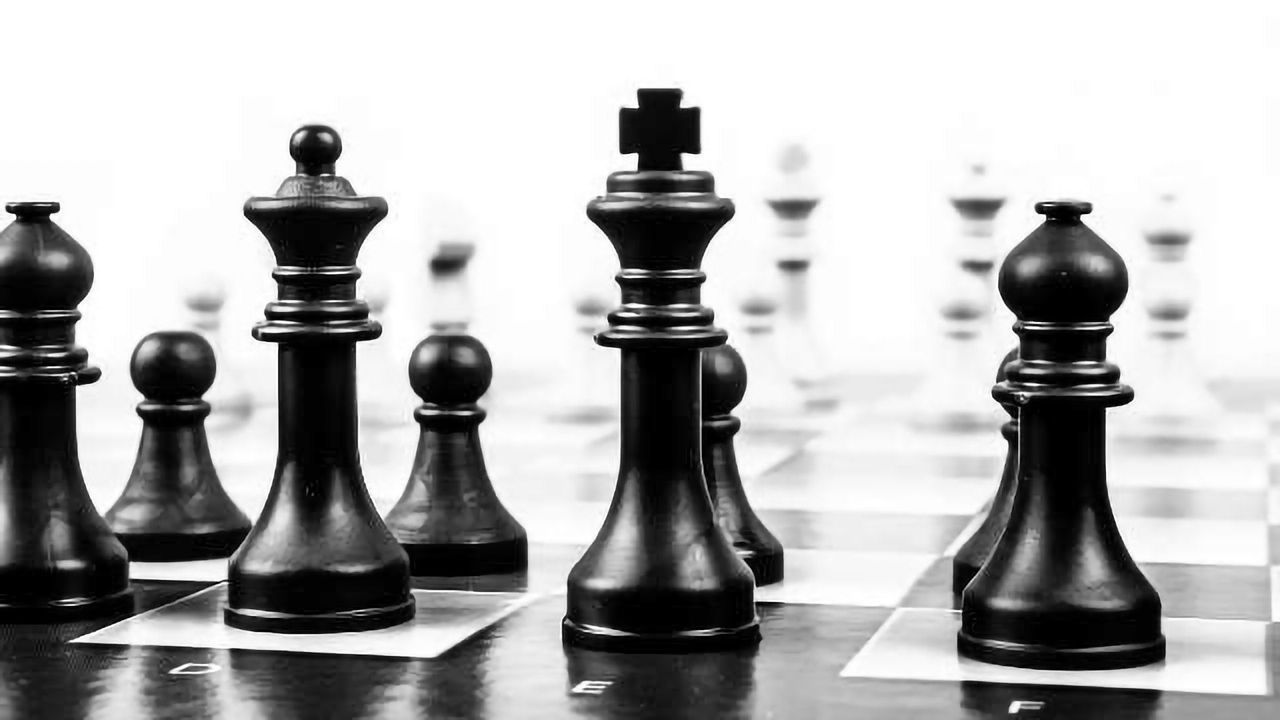
Although able to win against people, these engines relied largely on human knowledge of chess as encoded by expert programmers. A quarter century ago, the first engines appeared that were able to outplay world champions, most famously DeepBlue ( 2). Chess has been a testing ground for artificial intelligence since the time of Alan Turing ( 1).
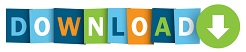